4. Examples
The following examples are available in a checkout of the repository
in the examples/
directory.
4.1. MCMC
'''This example illustrates how to run a Markov Chain using pypmc'''
import numpy as np
import pypmc
# define a proposal
prop_dof = 1.
prop_sigma = np.array([[0.1 , 0. ]
,[0. , 0.02]])
prop = pypmc.density.student_t.LocalStudentT(prop_sigma, prop_dof)
# define the target; i.e., the function you want to sample from.
# In this case, it is a Gaussian with mean "target_mean" and
# covariance "target_sigma".
#
# Note that the target function "log_target" returns the log of the
# unnormalized gaussian density.
target_sigma = np.array([[0.01 , 0.003 ]
,[0.003, 0.0025]])
inv_target_sigma = np.linalg.inv(target_sigma)
target_mean = np.array([4.3, 1.1])
def unnormalized_log_pdf_gauss(x, mu, inv_sigma):
diff = x - mu
return -0.5 * diff.dot(inv_sigma).dot(diff)
log_target = lambda x: unnormalized_log_pdf_gauss(x, target_mean, inv_target_sigma)
# choose a bad initialization
start = np.array([-2., 10.])
# define the markov chain object
mc = pypmc.sampler.markov_chain.AdaptiveMarkovChain(log_target, prop, start)
# run burn-in
mc.run(10**4)
# delete burn-in from samples
mc.clear()
# run 100,000 steps adapting the proposal every 500 steps
# hereby save the accept count which is returned by mc.run
accept_count = 0
for i in range(200):
accept_count += mc.run(500)
mc.adapt()
# extract a reference to the history of all visited points
values = mc.samples[:]
accept_rate = float(accept_count) / len(values)
print("The chain accepted %4.2f%% of the proposed points" % (accept_rate * 100) )
# plot the result
try:
import matplotlib.pyplot as plt
except ImportError:
print('For plotting "matplotlib" needs to be installed')
exit(1)
plt.hexbin(values[:,0], values[:,1], gridsize = 40, cmap='gray_r')
plt.show()
(Source code
, png
, hires.png
, pdf
)
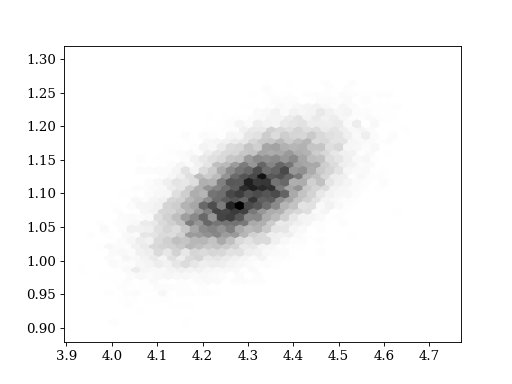
4.2. PMC
4.2.1. Serial
'''This example shows how to use importance sampling and how to
adapt the proposal density using the pmc algorithm.
'''
import numpy as np
import pypmc
# define the target; i.e., the function you want to sample from.
# In this case, it is a bimodal Gaussian
#
# Note that the target function "log_target" returns the log of the
# target function.
component_weights = np.array([0.3, 0.7])
mean0 = np.array ([ 5.0 , 0.01 ])
covariance0 = np.array([[ 0.01 , 0.003 ],
[ 0.003, 0.0025]])
inv_covariance0 = np.linalg.inv(covariance0)
mean1 = np.array ([-4.0 , 1.0 ])
covariance1 = np.array([[ 0.1 , 0. ],
[ 0. , 0.02 ]])
inv_covariance1 = np.linalg.inv(covariance1)
component_means = [mean0, mean1]
component_covariances = [covariance0, covariance1]
target_mixture = pypmc.density.mixture.create_gaussian_mixture(component_means, component_covariances, component_weights)
log_target = target_mixture.evaluate
# define the initial proposal density
# In this case a three-modal gaussian used
# the initial covariances are set to the unit-matrix
# the initial component weights are set equal
initial_prop_means = []
initial_prop_means.append( np.array([ 4.0, 0.0]) )
initial_prop_means.append( np.array([-5.0, 0.0]) )
initial_prop_means.append( np.array([ 0.0, 0.0]) )
initial_prop_covariance = np.eye(2)
initial_prop_components = []
for i in range(3):
initial_prop_components.append(pypmc.density.gauss.Gauss(initial_prop_means[i], initial_prop_covariance))
initial_proposal = pypmc.density.mixture.MixtureDensity(initial_prop_components)
# define an ImportanceSampler object
sampler = pypmc.sampler.importance_sampling.ImportanceSampler(log_target, initial_proposal)
# draw 10,000 samples adapting the proposal every 1,000 samples
# hereby save the generating proposal component for each sample which is
# returned by sampler.run
# Note: With too few samples components may die out, and one mode might be lost.
generating_components = []
for i in range(10):
print("\rstep", i, "...\n\t", end='')
# draw 1,000 samples and save the generating component
generating_components.append(sampler.run(10**3, trace_sort=True))
# get a reference to the weights and samples that have just been generated
samples = sampler.samples[-1]
weights = sampler.weights[-1][:,0]
# update the proposal using the pmc algorithm in the non Rao-Blackwellized form
pypmc.mix_adapt.pmc.gaussian_pmc(samples, sampler.proposal, weights, generating_components[-1],
mincount=20, rb=True, copy=False)
print("\rsampling finished")
print( '-----------------')
print('\n')
# print information about the adapted proposal
print('initial component weights:', initial_proposal.weights)
print('final component weights:', sampler.proposal.weights)
print('target component weights:', component_weights)
print()
for k, m in enumerate([mean0, mean1, None]):
print('initial mean of component %i:' %k, initial_proposal.components[k].mu)
print('final mean of component %i:' %k, sampler.proposal.components[k].mu)
print('target mean of component %i:' %k, m)
print()
print()
for k, c in enumerate([covariance0, covariance1, None]):
print('initial covariance of component %i:\n' %k, initial_proposal.components[k].sigma, sep='')
print()
print('final covariance of component %i:\n' %k, sampler.proposal.components[k].sigma, sep='')
print()
print('target covariance of component %i:\n' %k, c, sep='')
print('\n')
# plot results
try:
import matplotlib.pyplot as plt
except ImportError:
print('For plotting "matplotlib" needs to be installed')
exit(1)
def set_axlimits():
plt.xlim(-6.0, +6.000)
plt.ylim(-0.2, +1.401)
plt.subplot(221)
plt.title('target mixture')
pypmc.tools.plot_mixture(target_mixture, cmap='jet')
set_axlimits()
plt.subplot(222)
plt.title('pmc fit')
pypmc.tools.plot_mixture(sampler.proposal, cmap='nipy_spectral', cutoff=0.01)
set_axlimits()
plt.subplot(223)
plt.title('target mixture and pmc fit')
pypmc.tools.plot_mixture(target_mixture, cmap='jet')
pypmc.tools.plot_mixture(sampler.proposal, cmap='nipy_spectral', cutoff=0.01)
set_axlimits()
plt.subplot(224)
plt.title('weighted samples')
plt.hist2d(sampler.samples[-1][:,0], sampler.samples[-1][:,1], weights=sampler.weights[-1][:,0], cmap='gray_r', bins=200)
set_axlimits()
plt.tight_layout()
plt.show()
(Source code
, png
, hires.png
, pdf
)
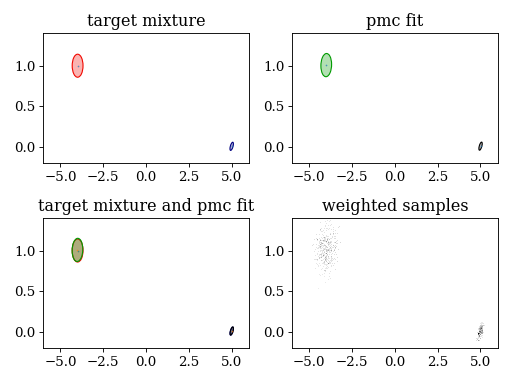
4.2.2. Parallel
'''This example shows how to use importance sampling and how to
adapt the proposal density using the pmc algorithm in an MPI
parallel environment.
In order to have a multiprocessing enviroment invoke this script with
"mpirun -n 10 python pmc_mpi.py".
'''
from mpi4py.MPI import COMM_WORLD as comm
import numpy as np
import pypmc
import pypmc.tools.parallel_sampler # this submodule is NOT imported by ``import pypmc``
# This script is a parallelized version of the PMC example ``pmc.py``.
# The following lines just define a target density and an initial proposal.
# These steps are exactly the same as in ``pmc.py``:
# define the target; i.e., the function you want to sample from.
# In this case, it is a bimodal Gaussian
#
# Note that the target function "log_target" returns the log of the
# target function.
component_weights = np.array([0.3, 0.7])
mean0 = np.array ([ 5.0 , 0.01 ])
covariance0 = np.array([[ 0.01 , 0.003 ],
[ 0.003, 0.0025]])
inv_covariance0 = np.linalg.inv(covariance0)
mean1 = np.array ([-4.0 , 1.0 ])
covariance1 = np.array([[ 0.1 , 0. ],
[ 0. , 0.02 ]])
inv_covariance1 = np.linalg.inv(covariance1)
component_means = [mean0, mean1]
component_covariances = [covariance0, covariance1]
target_mixture = pypmc.density.mixture.create_gaussian_mixture(component_means, component_covariances, component_weights)
log_target = target_mixture.evaluate
# define the initial proposal density
# In this case it has three Gaussians:
# the initial covariances are set to the unit-matrix,
# the initial component weights are set equal
initial_prop_means = []
initial_prop_means.append( np.array([ 4.0, 0.0]) )
initial_prop_means.append( np.array([-5.0, 0.0]) )
initial_prop_means.append( np.array([ 0.0, 0.0]) )
initial_prop_covariance = np.eye(2)
initial_prop_components = []
for i in range(3):
initial_prop_components.append(pypmc.density.gauss.Gauss(initial_prop_means[i], initial_prop_covariance))
initial_proposal = pypmc.density.mixture.MixtureDensity(initial_prop_components)
# -----------------------------------------------------------------------------------------------------------------------
# In ``pmc.py`` the following line defines the sequential single process sampler:
# sampler = pypmc.sampler.importance_sampling.ImportanceSampler(log_target, initial_proposal)
#
# We now use the parallel MPISampler instead:
SequentialIS = pypmc.sampler.importance_sampling.ImportanceSampler
parallel_sampler = pypmc.tools.parallel_sampler.MPISampler(SequentialIS, target=log_target, proposal=initial_proposal)
# Draw 10,000 samples adapting the proposal every 1,000 samples:
# make sure that every process has a different random number generator seed
if comm.Get_rank() == 0:
seed = np.random.randint(1e5)
else:
seed = None
seed = comm.bcast(seed)
np.random.seed(seed + comm.Get_rank())
generating_components = []
for i in range(10):
# With the invocation "mpirun -n 10 python pmc_mpi.py", there are
# 10 processes which means in order to draw 1,000 samples
# ``parallel_sampler.run(1000//comm.Get_size())`` makes each process draw
# 100 samples.
# Hereby the generating proposal component for each sample in each process
# is returned by ``parallel_sampler.run``.
# In the master process, ``parallel_sampler.run`` is a list containing the
# return values of the sequential ``run`` method of every process.
# In all other processes, ``parallel_sampler.run`` returns the generating
# component for its own samples only.
last_generating_components = parallel_sampler.run(1000//comm.Get_size(), trace_sort=True)
# In addition to the generating components, the ``sampler.run``
# method automatically sends all samples to the master
# process i.e. the process which fulfills comm.Get_rank() == 0.
if comm.Get_rank() == 0:
print("\rstep", i, "...\n\t", end='')
# Now let PMC run only in the master process:
# ``sampler.samples_list`` and ``sampler.weights_list`` store the weighted samples
# sorted by the resposible process:
# The History objects that are held by process i can be accessed via
# ``sampler.<samples/weights>_list[i]``. The master process (i=0) also produces samples.
# Combine the weights and samples to two arrays of 1,000 samples
samples = np.vstack([history_item[-1] for history_item in parallel_sampler.samples_list])
weights = np.vstack([history_item[-1] for history_item in parallel_sampler.weights_list])[:,0]
# The latent variables are stored in ``last_generating_components``.
# ``last_generating_components[i]`` returns an array with the generating
# components of the samples produced by process number "i".
# ``np.hstack(last_generating_components)`` combines the generating components
# from all processes to one array holding all 1,000 entries.
generating_components.append( np.hstack(last_generating_components) )
# adapt the proposal using the samples from all processes
new_proposal = pypmc.mix_adapt.pmc.gaussian_pmc(samples, parallel_sampler.sampler.proposal,
weights, generating_components[-1],
mincount=20, rb=True)
else:
# In order to broadcast the ``new_proposal``, define a dummy variable in the other processes
# see "MPI4Py tutorial", section "Collective Communication": http://mpi4py.scipy.org/docs/usrman/tutorial.html
new_proposal = None
# broadcast the ``new_proposal``
new_proposal = comm.bcast(new_proposal)
# replace the old proposal
parallel_sampler.sampler.proposal = new_proposal
# only the master process shall print out any final information
if comm.Get_rank() == 0:
all_samples = np.vstack([history_item[ :] for history_item in parallel_sampler.samples_list])
all_weights = np.vstack([history_item[ :] for history_item in parallel_sampler.weights_list])
last_samples = np.vstack([history_item[-1] for history_item in parallel_sampler.samples_list])
last_weights = np.vstack([history_item[-1] for history_item in parallel_sampler.weights_list])
print("\rsampling finished", end=', ')
print("collected " + str(len(all_samples)) + " samples")
print( '------------------------------------------')
print('\n')
# print information about the adapted proposal
print('initial component weights:', initial_proposal.weights)
print('final component weights:', parallel_sampler.sampler.proposal.weights)
print('target component weights:', component_weights)
print()
for k, m in enumerate([mean0, mean1, None]):
print('initial mean of component %i:' %k, initial_proposal.components[k].mu)
print('final mean of component %i:' %k, parallel_sampler.sampler.proposal.components[k].mu)
print('target mean of component %i:' %k, m)
print()
print()
for k, c in enumerate([covariance0, covariance1, None]):
print('initial covariance of component %i:\n' %k, initial_proposal.components[k].sigma, sep='')
print()
print('final covariance of component %i:\n' %k, parallel_sampler.sampler.proposal.components[k].sigma, sep='')
print()
print('target covariance of component %i:\n' %k, c, sep='')
print('\n')
if comm.Get_size() == 1:
print('******************************************************')
print('********** NOTE: There is only one process. **********')
print('******** try "mpirun -n 10 python pmc_mpi.py" ********')
print('******************************************************')
# plot results
try:
import matplotlib.pyplot as plt
except ImportError:
print('For plotting "matplotlib" needs to be installed')
exit(1)
def set_axlimits():
plt.xlim(-6.0, +6.000)
plt.ylim(-0.2, +1.401)
plt.subplot(221)
plt.title('target mixture')
pypmc.tools.plot_mixture(target_mixture, cmap='jet')
set_axlimits()
plt.subplot(222)
plt.title('pmc fit')
pypmc.tools.plot_mixture(parallel_sampler.sampler.proposal, cmap='nipy_spectral', cutoff=0.01)
set_axlimits()
plt.subplot(223)
plt.title('target mixture and pmc fit')
pypmc.tools.plot_mixture(target_mixture, cmap='jet')
pypmc.tools.plot_mixture(parallel_sampler.sampler.proposal, cmap='nipy_spectral', cutoff=0.01)
set_axlimits()
plt.subplot(224)
plt.title('weighted samples')
plt.hist2d(last_samples[:,0], last_samples[:,1], weights=last_weights[:,0], cmap='gray_r', bins=200)
set_axlimits()
plt.tight_layout()
plt.show()
(Source code
, png
, hires.png
, pdf
)
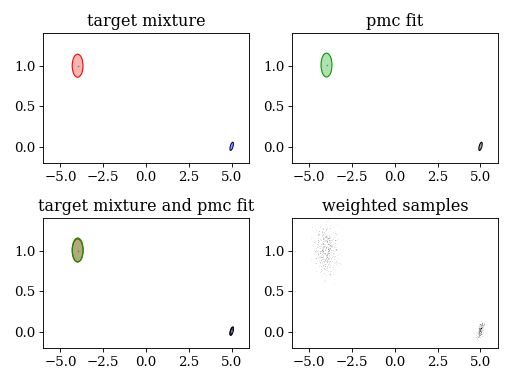
4.3. Grouping by Gelman-Rubin R value
'''This example illustrates how to group Markov Chains according to the
Gelman-Rubin R value (see [GR92]_).
'''
import numpy as np
import pypmc
# A Markov Chain can only explore a local mode of the target function.
# The Gelman-Rubin R value can be used to determine whether N chains
# explored the same mode. Pypmc offers a function which groups chains
# with a common R value less than some ``critical_r``.
#
# In this example, we run five Markov Chains initialized in different
# modes and then group those chains together that explored same mode.
# define a proposal
# this defines the same initial proposal for all chains
prop_dof = 50.
prop_sigma = np.array([[0.1 , 0. ]
,[0. , 0.02]])
prop = pypmc.density.student_t.LocalStudentT(prop_sigma, prop_dof)
# define the target; i.e., the function you want to sample from.
# In this case, it is a bimodal Gaussian with well separated modes.
#
# Note that the target function "log_target" returns the log of the
# target function.
component_weights = np.array([0.3, 0.7])
mean0 = np.array ([ 5.0 , 0.01 ])
covariance0 = np.array([[ 0.01 , 0.003 ],
[ 0.003, 0.0025]])
inv_covariance0 = np.linalg.inv(covariance0)
mean1 = np.array ([-4.0 , 1.0 ])
covariance1 = np.array([[ 0.1 , 0. ],
[ 0. , 0.02 ]])
inv_covariance1 = np.linalg.inv(covariance1)
component_means = [mean0, mean1]
component_covariances = [covariance0, covariance1]
target_mixture = pypmc.density.mixture.create_gaussian_mixture(component_means, component_covariances, component_weights)
log_target = target_mixture.evaluate
# choose initializations for the chains
# Here we place two chains into the mode at [5, 0.01] and three into the mode at [-4,1].
# In such a setup, the chains will only explore the mode where they are initialized.
# Different random numbers are used in each chain.
starts = [np.array([4.999, 0.])] * 2 + [np.array([-4.0001, 0.999])] * 3
# define the markov chain objects
mcs = [pypmc.sampler.markov_chain.AdaptiveMarkovChain(log_target, prop, start) for start in starts]
# run and discard burn-in
for mc in mcs:
mc.run(10**2)
mc.clear()
# run 10,000 steps adapting the proposal every 500 steps
for mc in mcs:
for i in range(20):
mc.run(500)
mc.adapt()
# extract a reference to the samples from all chains
stacked_values = [mc.samples[:] for mc in mcs]
# find the chain groups
# chains 0 and 1 are initialized in the same mode (at [5, 0.01])
# chains 2, 3 and 4 are initialized in the same mode (at [-4, 0])
# expect chain groups:
expected_groups = [[0,1], [2,3,4]]
# R value calculation only needs the means, variances (diagonal
# elements of covariance matrix) and number of samples,
# axis=0 ensures that we get variances separately for each parameter.
# critical_r can be set manually, here the default value is used
found_groups = pypmc.mix_adapt.r_value.r_group([np.mean(chain, axis=0) for chain in stacked_values],
[np.var (chain, axis=0) for chain in stacked_values],
len(stacked_values[0]))
# print the result
print("Expect %s" % expected_groups)
print("Have %s" % found_groups)
# Hint: ``stacked_values`` is an example of what `pypmc.mix_adapt.r_value.make_r_gaussmix()` expects as ``data``
result = pypmc.mix_adapt.r_value.make_r_gaussmix(stacked_values)
try:
import matplotlib.pyplot as plt
except ImportError:
print('For plotting "matplotlib" needs to be installed')
exit(1)
pypmc.tools.plot_mixture(result, cmap='jet')
plt.show()
(Source code
, png
, hires.png
, pdf
)
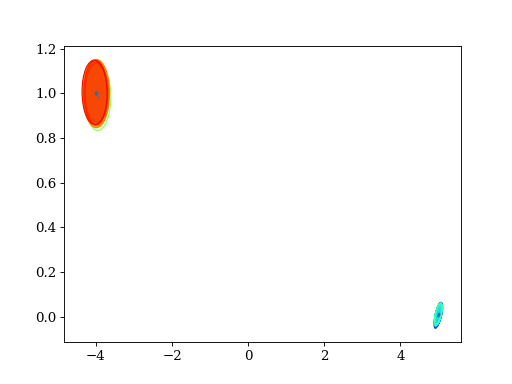
4.4. Variational Bayes
'''This example shows how to generate a "best fit" Gaussian mixture density
from data using variational Bayes.
'''
## in this example, we will:
## 1. Define a Gaussian mixture
## 2. Generate demo data from that Gaussian mixture
## 3. Generate a Gaussian mixture out of the data
## 4. Plot the original and the generated mixture
import numpy as np
import pypmc
# -------------------- 1. Define a Gaussian mixture --------------------
component_weights = np.array([0.3, 0.7])
mean0 = np.array ([ 5.0 , 0.01 ])
covariance0 = np.array([[ 0.01 , 0.003 ],
[ 0.003, 0.0025]])
mean1 = np.array ([-4.0 , 1.0 ])
covariance1 = np.array([[ 0.1 , 0. ],
[ 0. , 0.02 ]])
component_means = [mean0, mean1]
component_covariances = [covariance0, covariance1]
target_mix = pypmc.density.mixture.create_gaussian_mixture(component_means, component_covariances, component_weights)
# -------------------- 2. Generate demo data ---------------------------
data = target_mix.propose(500)
# -------------------- 3. Adapt a Gaussian mixture ---------------------
# maximum number of components
K = 20
# Create a "GaussianInference" object.
# The following command passes just the two essential arguments to "GaussianInference":
# The ``data`` and a maximum number of ``components``.
# For reasonable results in more complicated settings, a careful choice for ``W0``
# is crucial. As a rule of thumb, choose ``inv(W0)`` much smaller than the expected
# covariance. In this case, however, the default (``W0`` = unit matrix) is good enough.
vb = pypmc.mix_adapt.variational.GaussianInference(data, K)
# adapt the variational parameters
converged = vb.run(100, verbose=True)
print('-----------------------------')
# generate a Gaussian mixture with the most probable parameters
fit_mixture = vb.make_mixture()
# -------------------- 4. Plot/print results ---------------------------
if converged is None:
print('\nThe adaptation did not converge.\n')
else:
print('\nConverged after %i iterations.\n' %converged)
print("final component weights: " + str(fit_mixture.weights))
print("target component weights: " + str( target_mix.weights))
try:
import matplotlib.pyplot as plt
except ImportError:
print('For plotting "matplotlib" needs to be installed')
exit(1)
def set_axlimits():
plt.xlim(-6.0, +6.000)
plt.ylim(-0.2, +1.401)
plt.subplot(221)
plt.title('target mixture')
pypmc.tools.plot_mixture(target_mix, cmap='winter')
set_axlimits()
plt.subplot(222)
plt.title('"best fit"')
pypmc.tools.plot_mixture(fit_mixture, cmap='nipy_spectral')
set_axlimits()
plt.subplot(223)
plt.title('target mixture and "best fit"')
pypmc.tools.plot_mixture(target_mix, cmap='winter')
pypmc.tools.plot_mixture(fit_mixture, cmap='nipy_spectral')
set_axlimits()
plt.subplot(224)
plt.title('data')
plt.hexbin(data[:,0], data[:,1], cmap='gray_r')
set_axlimits()
plt.tight_layout()
plt.show()
(Source code
, png
, hires.png
, pdf
)
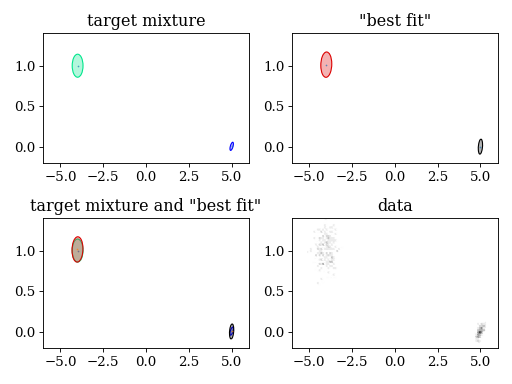
4.5. Mixture reduction
'''Demonstrate the usage of hierarchical clustering and variational
Bayes (VBMerge) to reduce a given Gaussian mixture to a Gaussian
mixture with a reduced number of components.
'''
import numpy as np
from scipy.stats import chi2
import pypmc
# dimension
D = 2
# number of components
K = 400
# Wishart parameters: mean W, degree of freedom nu
W = np.eye(D)
nu = 5
# "draw" covariance matrices from Wishart distribution
def wishart(nu, W):
dim = W.shape[0]
chol = np.linalg.cholesky(W)
tmp = np.zeros((dim,dim))
for i in range(dim):
for j in range(i+1):
if i == j:
tmp[i,j] = np.sqrt(chi2.rvs(nu-(i+1)+1))
else:
tmp[i,j] = np.random.normal(0,1)
return np.dot(chol, np.dot(tmp, np.dot(tmp.T, chol.T)))
covariances = [wishart(nu, W) for k in range(K)]
# put components at positions drawn from a Gaussian around mu
mu = np.zeros(D)
means = [np.random.multivariate_normal(mu, sigma) for sigma in covariances]
# equal weights for every component
weights = np.ones(K)
# weights are automatically normalized
input_mixture = pypmc.density.mixture.create_gaussian_mixture(means, covariances, weights)
# create initial guess from first K_out components
K_out = 10
initial_guess = pypmc.density.mixture.create_gaussian_mixture(means[:K_out], covariances[:K_out], weights[:K_out])
###
# hierarchical clustering
#
# - the output closely resembles the initial guess
# - components laid out spherically symmetric
# - every component is preserved
###
h = pypmc.mix_adapt.hierarchical.Hierarchical(input_mixture, initial_guess)
h.run(verbose=True)
###
# VBMerge
#
# - N is the number of samples that gave rise to the input mixture. It
# is arbitrary, so play around with it. You might have to adjust the
# ``prune`` parameter in the ``run()`` method
# - only one component survives, again it is spherically symmetric
###
vb = pypmc.mix_adapt.variational.VBMerge(input_mixture, N=1000,
initial_guess=initial_guess)
print()
print("Start variational Bayes:")
vb.run(verbose=True)
# plot results
try:
import matplotlib.pyplot as plt
except ImportError:
print('For plotting "matplotlib" needs to be installed')
exit(1)
def set_axlimits():
plt.gca().set_aspect('equal')
plt.xlim(-12.0, +12.0)
plt.ylim(-12.0, +12.0)
plt.subplot(221)
plt.title('input mixture')
pypmc.tools.plot_mixture(input_mixture)
set_axlimits()
plt.subplot(222)
plt.title('initial guess')
pypmc.tools.plot_mixture(initial_guess)
set_axlimits()
plt.subplot(223)
plt.title('variational Bayes')
pypmc.tools.plot_mixture(vb.make_mixture(), cmap='autumn')
set_axlimits()
plt.subplot(224)
plt.title('hierarchical output')
pypmc.tools.plot_mixture(h.g)
set_axlimits()
plt.tight_layout()
plt.show()
(Source code
, png
, hires.png
, pdf
)
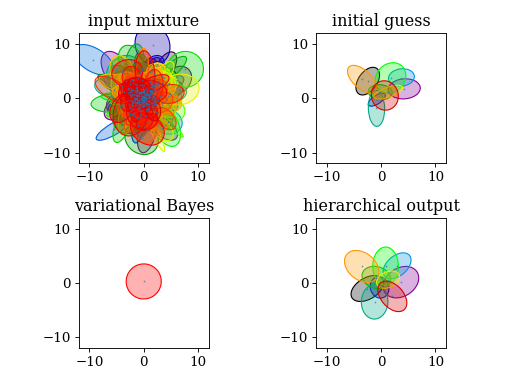
4.6. MCMC + variational Bayes
'''This example illustrates how pypmc can be used to integrate a
non-negative function. The presented algorithm needs very little
analytical knowledge about the function.
'''
import numpy as np
import pypmc
# The idea is to find a good proposal function for importance sampling
# with as little information about the target function as possible.
#
# In this example we will first map out regions of interest using Markov
# chains, then we use the variational Bayes to approximate the target
# with a Gaussian mixture.
# *************************** Important: ***************************
# * The target function must be defined such that it returns the *
# * log of the function of interest. The methods we use imply that *
# * the function is interpreted as an unnormalized probability *
# * density. *
# ******************************************************************
# Define the target; i.e., the function you want to sample from. In
# this case, it is a Student's t mixture of three components with
# different degrees of freedom. They are located close to each other.
# If you want a multimodal target, adjust the means.
dim = 2
mean0 = np.array ([-6.0, 7.3 ])
covariance0 = np.array([[ 0.8, -0.3 ],
[-0.3, 1.25]])
mean1 = np.array ([-7.0, 8.0 ])
covariance1 = np.array([[ 0.5, 0. ],
[ 0. , 0.2 ]])
mean2 = np.array ([-8.5, 7.5 ])
covariance2 = np.array([[ 0.5, 0.2 ],
[ 0.2, 0.2 ]])
component_weights = np.array([0.3, 0.4, 0.3])
component_means = [mean0, mean1, mean2]
component_covariances = [covariance0, covariance1, covariance2]
dofs = [13, 17, 5]
target_mixture = pypmc.density.mixture.create_t_mixture(component_means, component_covariances, dofs, component_weights)
log_target = target_mixture.evaluate
# Now we suppose that we only have the following knowledge about the
# target function: its regions of interest are at a distance of no more
# than order ten from zero.
# Now we try to find these with Markov chains. We have to deal with
# the fact that there may be modes separated by regions of very low
# probability. It is thus unlikely that a single chain explores more
# than one mode in such a case. To deal with this multimodality, we
# start several chains and hope that they find all modes. We will
# start ten Markov chains at random positions in the square
# [(-10,-10), (+10,+10)].
starts = [np.random.uniform(-10,10, size=dim) for i in range(10)]
# For a local-random-walk Markov chain, we also need an initial
# proposal. Here, we take a gaussian with initial covariance
# diag(1e-3). The initial covariance should be chosen such that it is
# of the same order as the real covariance of the mode to be mapped
# out. For a Gaussian target, the overall scale should
# decrease as 2.38^2/d as the dimension d increases to achieve an
# acceptance rate around 20%.
mc_prop = pypmc.density.gauss.LocalGauss(np.eye(dim) * 2.38**2 / dim)
mcs = [pypmc.sampler.markov_chain.AdaptiveMarkovChain(log_target, mc_prop, start) for start in starts]
print('running Markov chains ...')
# In general we need to let the chain move to regions of high
# probability, these samples are not representative, so we discard them
# as burn-in. Then we let the Markov chains map out the regions of
# interest. The samples are used to adapt the proposal covariance to
# yield a satisfactory acceptance rate.
for mc in mcs:
for i in range(20):
mc.run(500)
mc.adapt()
if i == 0:
mc.clear()
mc_samples_sorted_by_chain = [mc.samples[:] for mc in mcs]
mc_samples = np.vstack(mc_samples_sorted_by_chain)
means = np.zeros((len(mcs), dim))
variances = np.zeros_like(means)
for i,mc in enumerate(mc_samples_sorted_by_chain):
means[i] = mc.mean(axis=0)
variances[i] = mc.var(axis=0)
# Now we use the Markov chain samples to generate a mixture proposal
# function for importance sampling. For this purpose, we choose the
# variational Bayes algorithm that takes samples and an initial guess
# of the mixture as input. To create the initial guess, we group all
# chains that mixed, and create 10 components per group. For a
# unimodal target, all chains should mix. For more information about
# the following call, check the example "Grouping by Gelman-Rubin R
# value"(r-group.py) or the reference documentation.
long_patches = pypmc.mix_adapt.r_value.make_r_gaussmix(mc_samples_sorted_by_chain, K_g=10)
# Comments on arguments:
# o mc_samples[::100] - Samples in the Markov chains are strongly correlated
# => thin the samples to get approx. independent samples
# o W0=np.eye(dim)*1e10 - The resulting covariance matrices can be very
# sensitive to W0. Its inverse should be chosen much
# smaller than the actual covariance. If it is too small,
# W0 will dominate the resulting covariances and
# usually lead to very bad results.
vb = pypmc.mix_adapt.variational.GaussianInference(mc_samples[::100], initial_guess=long_patches, W0=np.eye(dim)*1e10)
# When we run variational Bayes, we want unneccessary components to be
# automatically pruned. The prune parameter sets how many samples a
# component must effectively have to be considered important. The rule
# of thumb employed here proved good in our experiments.
vb_prune = 0.5 * len(vb.data) / vb.K
# Run the variational Bayes for at most 1,000 iterations. But if the
# lower bound of the model evidence changes by less than `rel_tol`,
# convergence is declared before. If we increase `rel_tol` to 1e-4, it
# takes less iterations but potentially more (useless) components
# survive the pruning. The trade-off depends on the complexity of the
# problem.
print('running variational Bayes ...')
vb.run(1000, rel_tol=1e-8, abs_tol=1e-5, prune=vb_prune, verbose=True)
# extract the most probable Gaussian mixture given the samples
vbmix = vb.make_mixture()
# Now we can instantiate an importance sampler. We draw 1,000
# importance samples and use these for a proposal update using
# variational Bayes again. In case there are multiple modes and
# the chains did not mix, we need this step to infer the right
# component weights because the component weight is given by
# how many chains it attracted, which could be highly dependent
# on the starting points and independent of the correct
# probability mass.
print('running importance sampling ...')
sampler = pypmc.sampler.importance_sampling.ImportanceSampler(log_target, vbmix)
sampler.run(1000)
# The variational Bayes allows us, unlike PMC, to include the
# information gained by the Markov chains in subsequent proposal
# updates. We know that we cannot trust the component weights obtained
# by the chains. Nevertheless, we can rely on the means and covariances. The
# following lines show how to code that into the variational Bayes by
# removing the hyperparameter `alpha0` that encodes the component
# weights.
prior_for_proposal_update = vb.posterior2prior()
prior_for_proposal_update.pop('alpha0')
vb2 = pypmc.mix_adapt.variational.GaussianInference(sampler.samples[:],
initial_guess=vbmix,
weights=sampler.weights[:][:,0],
**prior_for_proposal_update)
# Note: This time we leave "prune" at the default value "1" because we
# want to keep all components that are expected to contribute
# with at least one effective sample per importance sampling run.
print('running variational Bayes ...')
vb2.run(1000, rel_tol=1e-8, abs_tol=1e-5, verbose=True)
vb2mix = vb2.make_mixture()
# Now we draw another 10,000 samples with the updated proposal
sampler.proposal = vb2mix
print('running importance sampling ...')
sampler.run(10**4)
# We can combine the samples and weights from the two runs, see reference [Cor+12].
weights = pypmc.sampler.importance_sampling.combine_weights([samples[:] for samples in sampler.samples],
[weights[:][:,0] for weights in sampler.weights],
[vbmix, vb2mix] ) \
[:][:,0]
samples = sampler.samples[:]
# The integral can then be estimated from the weights. The error is also
# estimated from the weights. By the central limit theorem, the integral
# estimator has a gaussian distribution.
integral_estimator = weights.sum() / len(weights)
integral_uncertainty_estimator = np.sqrt((weights**2).sum() / len(weights) - integral_estimator**2) / np.sqrt(len(weights)-1)
print('analytical integral = 1')
print('estimated integral =', integral_estimator, '+-', integral_uncertainty_estimator)
# Let's see how good the proposal matches the target density: the closer
# the values of perplexity and effective sample size (ESS) are to 1,
# the better. Outliers, or samples out in the tails of the target
# with a very large weight, show up in the 2D marginal and reduce the
# ESS significantly. For the above integral estimate to be right on
# average, they are 'needed'. Without outliers (most of the time), the
# integral is a tad too small.
print('perplexity', pypmc.tools.convergence.perp(weights))
print('effective sample size', pypmc.tools.convergence.ess(weights))
# As mentioned in the very beginning, the methods we applied reinterpret
# the target function as an unnormalized probability density.
# In addition to the integral, we also get weighted samples distributed according
# to that probability density.
try:
import matplotlib.pyplot as plt
except ImportError:
print('For plotting "matplotlib" needs to be installed')
exit(1)
plt.figure()
plt.hist2d(samples[:,0], samples[:,1], weights=weights, bins=100, cmap='gray_r')
pypmc.tools.plot_mixture(sampler.proposal, visualize_weights=True, cmap='jet')
plt.colorbar()
plt.clim(0.0, 1.0)
plt.title('colors visualize component weights')
plt.show()
(Source code
, png
, hires.png
, pdf
)
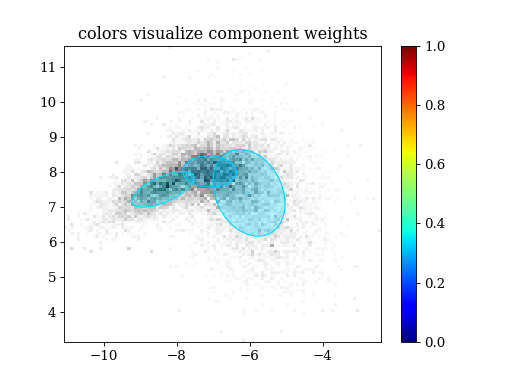